Deep learning for the detection and phase unwrapping of mining-induced deformation in large-scale interferograms
Zhipeng Wu; Teng Wang; Yingjie Wang; Robert Wang; Daqing Ge
Published in: IEEE Transactions on Geoscience and Remote Sensing, SCI, Top期刊, IF=5.6
Abstract:
This paper proposes deep convolutional neural networks to detect and map localized, rapid subsidence caused by mining activities using time-series Sentinel-1 SAR images. A deformation detection network (DDNet) is developed to automatically identify rapidly subsiding areas from wrapped interferograms, and a phase unwrapping network (PUNet) is designed to unwrap the cropped interferogram patches centered on the detected subsiding locations. To train the two networks, interferogram simulation strategies are developed to generate various training samples using the distorted two-dimensional Gaussian surface and fractal Perlin noises. The performance of the DDNet is verified by simulations on a synthetic dataset with 13 large interferograms, while the PUNet is evaluated by simulations using synthetic datasets with different levels of deformation gradients and noises. Compared with the traditional and deep-learning methods, the PUNet exhibits excellent performance and efficiency in unwrapping interferograms with rapid mining-induced deformation. The proposed networks are further verified by applying them to Shanxi Province, China, which is characterized by serious ground subsidence hazards caused by long-term coal mining activities. The time-series deformations of 1,344 detected subsidence areas are calculated with the vertical velocities ranging from -19.7 cm/year to -254.8 cm/year. The results are validated using the ascending and descending Sentinel-1 Interferograms and an L-band ALOS-2 interferogram covering the same area within the acquisition period, showing highly consistent vertical deformation rates. The proposed strategy and methods introduce deep learning to the time-series InSAR processing chain and may have profound implications on the detection and monitoring of localized mining-induced deformation using InSAR.
本文提出了利用深度卷积神经网络和时间序列哨兵-1 SAR图像对采矿活动引起的局部快速沉降进行自动化探测和时序分析的数据处理策略和方法。其中形变探测网络(DDNet)用于从缠绕的干涉图中自动识别快速沉降的区域;相位解缠网络(PUNet)用于解缠探测到的沉降位置为中心的干涉图切片。为了训练这两个网络,我们开发了一套沉降漏斗干涉图模拟方法,使用扭曲的二维高斯表面和分形Perlin噪声产生各种训练样本。DDNet 的性能通过在13 景模拟干涉图数据集上进行验证,而 PUNet 则通过使用具有不同形变梯度和噪声水平的模拟数据集来评估,并使用升降轨哨兵-1干涉图和覆盖同一地区的L波段ALOS-2干涉图实际验证了解缠效果。所提出的网络通过在中国山西省的应用得到了进一步验证,从2018年9月10日至2019年11月16日获取的哨兵-1数据中共探测到 1,344 个沉降漏斗区域,并获取它们的年度形变序列。其中最大垂直形变速率范围从 -19.7 厘米/年到 -254.8 厘米/年。与传统和其他深度学习解缠方法相比,PUNet 在解缠采矿引起的快速形变干涉图方面表现出优异的性能和效率。我们所提出的策略和方法将深度学习引入到时间序列InSAR处理链中,并对利用InSAR探测和监测局部形变异常提供了新的思路。
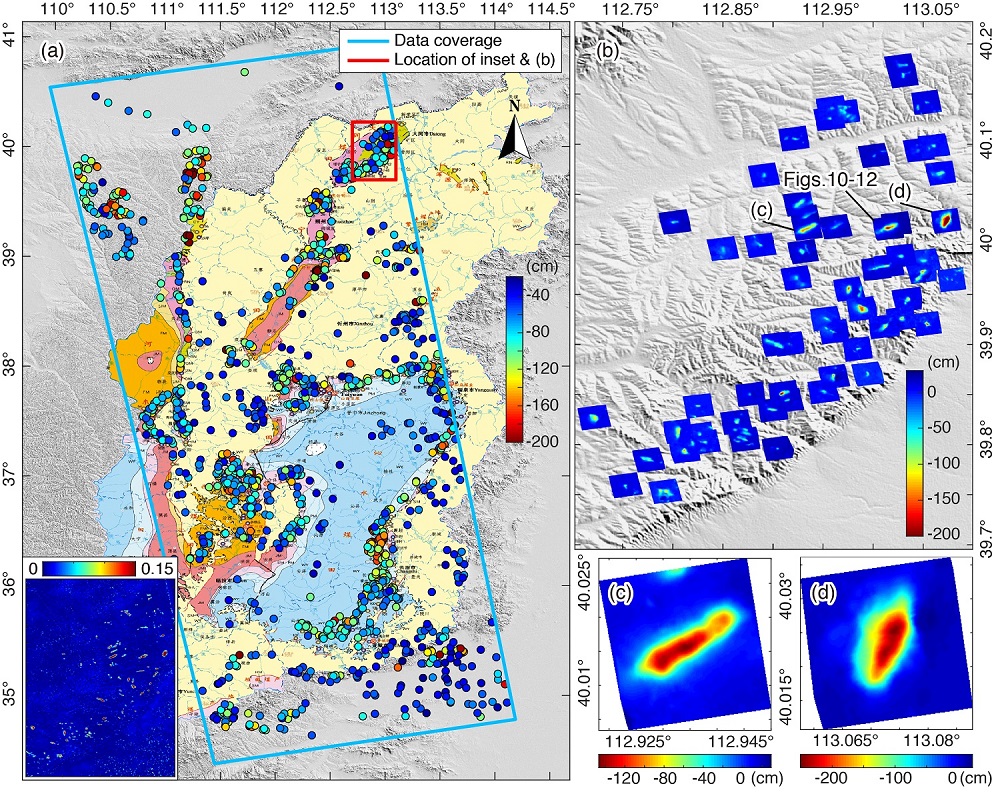
2018年9月10日至2019年11月16日山西省局部沉降区的监测结果,投影至垂直方向,其中负值,即红色,代表沉降。(a) 1344个探测到的沉降区叠加在山西省的煤炭资源分布图上。点的颜色表示最大累积沉降量。为了可视化,所有小于-200厘米的点都被设置为与-200厘米相同的颜色。插图显示的是DDNet在36张干涉图上得到的雷达坐标系的平均归一化形变。(b)中显示了分布在(a)中红色矩形区域内的累积沉降量,而(c)和(d)中显示了两个局部沉降区域的细节。
Citation
Z. Wu, T. Wang, Y. Wang, R. Wang and D. Ge, “Deep learning for the detection and phase unwrapping of mining-induced deformation in large-scale interferograms,” in IEEE Transactions on Geoscience and Remote Sensing, doi: 10.1109/TGRS.2021.3121907.
Authors
Zhipeng Wu (Department of Space Microwave Remote Sensing System, Aerospace Information Research Institute, Chinese Academy of Sciences, Beijing 100190, China, and School of Electronic, Electrical, and Communication Engineering, University of Chinese Academy of Sciences, Beijing 100039, China.)
Teng Wang (School of Earth and Space Sciences, Peking University, Beijing 100871, China.)
Yingjie Wang (Department of Space Microwave remote Sensing System, Aerospace Information Research Institute, Chinese Academy of Sciences, Beijing 100190, China.)
Robert Wang (Department of Space Microwave Remote Sensing System, Aerospace Information Research Institute, Chinese Academy of Sciences, Beijing 100190, China, and School of Electronic, Electrical, and Communication Engineering, University of Chinese Academy of Sciences, Beijing 100039, China.)
Daqing Ge (China Aero Geophysical Survey & Remote Sensing Center for Land and Resources (AGRS), Beijing 100083, China.)