Deep-learning based phase discontinuity prediction for two-dimensional phase unwrapping of SAR interferograms
Zhipeng Wu; Teng Wang; Yingjie Wang; Robert Wang; Daqing Ge
Published in: IEEE Transactions on Geoscience and Remote Sensing, SCI, Top期刊, IF=5.6
Abstract:
Phase unwrapping is a critical step of interferometric synthetic aperture radar (InSAR) processing, and its accuracy directly determines the reliability of subsequent applications. Many phase unwrapping methods have been proposed, most of which assume that the phase has spatial continuity, while decorrelation noise and aliasing fringes invalidate the assumptions, resulting in poor performance of these methods. To obtain more reliable unwrapping results, in this paper, a deep convolutional neural network, called DENet, is proposed for predicting the probabilities of phase discontinuities in interferograms. The main advantages of DENet are: (1) using branching structure to extract detailed and high-level features separately, to retain details while making full use of contextual information; (2) using multi-channel input, including interferogram, range/azimuthal phase gradients, and residues map, to provide effective guidance for discontinuity prediction; and (3) using a single network to estimate phase discontinuities in both range and azimuth directions simultaneously. To train the network, a data set simulation strategy is proposed to generate enough training samples. The strategy considers a variety of phase components such as terrain-related phase, random deformation, atmospheric turbulence, and noise. The phase discontinuity estimated by DENet is then converted to costs in the minimum cost flow solver of SNAPHU to obtain the final unwrapped phase. Based on validations of simulated and real interferograms, the proposed method exhibits excellent performance compared to traditional and deep learning unwrapping methods. The proposed method can effectively unwrap large-scale, low-quality interferograms, which is expected to significantly improve the accuracy of InSAR applications.
相位解缠是合成孔径雷达干涉测量(InSAR)数据处理的一个关键步骤,其准确性直接决定了后续应用的可靠性。许多相位解缠方法已被提出,其中大多数方法假设相位具有空间连续性,而去相关噪声和混叠条纹使该假设失效。当无法准确判断发生相位不连续的位置时,很多方法的解缠性能会显著下降。基于此问题,本文提出了一个深度卷积神经网络:DENet,用于预测干涉图中所有位置出现相位不连续的概率,用于辅助传统的最小费用流解缠算法。DENet的主要优点是:(1)利用分支结构分别提取细节和高层特征,在保留细节的同时充分利用上下文信息;(2)利用多通道输入,包括干涉图、距离向/方位向相位梯度和残数图,为不连续预测提供有效指导;(3)利用单一网络同时估计距离向和方位向的相位不连续。为了训练网络,我们提出了一个数据集模拟策略来生成足够的训练样本。该策略考虑了各种相位成分,如与地形有关的相位、随机形变、大气湍流和噪声。然后,由DENet估计的相位不连续被转换为SNAPHU中最小费用流求解器中的费用,用于获得最终的解缠相位。基于对模拟和真实干涉图的验证,与传统和深度学习解缠方法相比,本文所提出的方法表现出优异的性能,可以有效地解缠大尺度、低质量、复杂条纹分布的干涉图,有望显著扩展InSAR应用的领域。
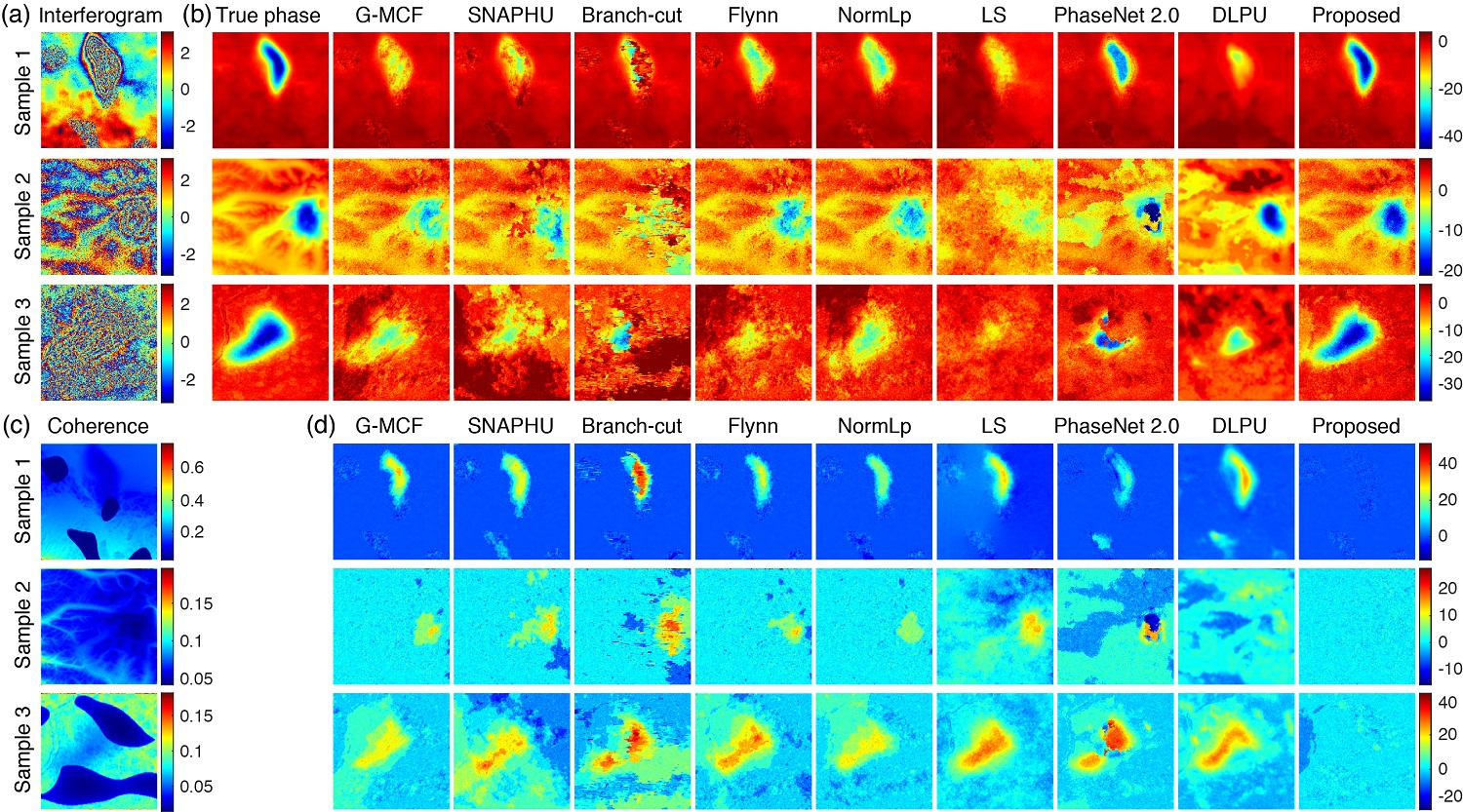
大尺度干涉图上不同方法的解缠结果。(a)显示的是2019年5月12日至7月23日期间获取的包含五个标准幅的降轨Sentinel-1干涉图,其覆盖面积约为860公里×255公里。干涉图中存在着明显的大气延迟相位,同时被完全失相干的水域所分割。这些区域在相干图中表现为低值,如(b)所示。本文提出的方法被应用于解缠这种大规模的干涉图,并将其结果与参考方法进行了比较,如(c)-(h)所示。其中G-MCF,G-MCF patching和G-MCF masking分别为应用GAMMA软件中的MCF解缠方法,用该方法的分块模式和加入掩膜模式进行解缠的结果。
Citation
Z. Wu, T. Wang, Y. Wang, R. Wang and D. Ge, “Deep-learning based phase discontinuity prediction for two-dimensional phase unwrapping of SAR interferograms,” in IEEE Transactions on Geoscience and Remote Sensing, doi: 10.1109/TGRS.2021.3121906.
Authors
Zhipeng Wu (Department of Space Microwave Remote Sensing System, Aerospace Information Research Institute, Chinese Academy of Sciences, Beijing 100190, China, and School of Electronic, Electrical, and Communication Engineering, University of Chinese Academy of Sciences, Beijing 100039, China.)
Teng Wang (School of Earth and Space Sciences, Peking University, Beijing 100871, China.)
Yingjie Wang (Department of Space Microwave remote Sensing System, Aerospace Information Research Institute, Chinese Academy of Sciences, Beijing 100190, China.)
Robert Wang (Department of Space Microwave Remote Sensing System, Aerospace Information Research Institute, Chinese Academy of Sciences, Beijing 100190, China, and School of Electronic, Electrical, and Communication Engineering, University of Chinese Academy of Sciences, Beijing 100039, China.)
Daqing Ge (China Aero Geophysical Survey & Remote Sensing Center for Land and Resources (AGRS), Beijing 100083, China.)